Predictive Analytics: What, Why, Who, and How? by DataDrivify
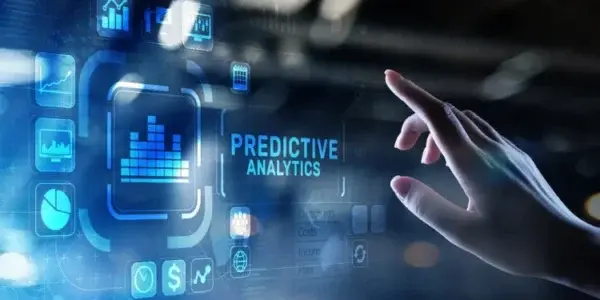
1. What is Predictive Analytics
Predictive Analytics is a branch of advanced analytics that involves the use of data, statistical algorithms, and machine learning techniques to forecast future outcomes. Leveraging historical and current data, it seeks to anticipate the probability of future events by identifying patterns and trends. This forward-looking perspective is what sets predictive analytics apart from other forms of business intelligence and analytics.
2. Purpose and What does it Solve
Predictive Analytics aims to assist organizations in making informed and proactive decisions. It turns raw data into actionable insights, helping to reduce risk, optimize operations, and uncover new opportunities. Some areas where predictive analytics provides solutions include:
Risk Management: Predictive analytics helps organizations identify potential risks and mitigate them proactively. For instance, credit scoring used by financial institutions to assess the likelihood of loan default is a classic example of risk management with predictive analytics.
Operational Efficiency: By predicting demand, organizations can optimize resource allocation, inventory management, and production planning, leading to increased operational efficiency.
Customer Retention: Predictive models can forecast customer churn, allowing businesses to develop strategies that improve customer retention.
3. Who / Which Industry Should Adopt Predictive Analytics
Predictive analytics is beneficial across a myriad of sectors. Some prominent ones include:
Healthcare: Predictive analytics can forecast disease outbreaks, improve patient care, and optimize hospital resource allocation.
Retail: It aids in predicting customer purchasing behavior, optimizing inventory, and personalizing marketing efforts.
Financial Services: It’s used in credit scoring, fraud detection, and investment predictions.
Manufacturing: Predictive maintenance models help predict equipment failures and schedule maintenance, thus reducing downtime.
Transportation: It assists in optimizing routes, forecasting demand, and managing fleet effectively.
4. Inputs Considered for the Model
Predictive analytics relies heavily on accurate and relevant data. These data inputs typically include:
Historical Data: This forms the backbone of predictive modeling. It includes past transactions, behaviors, and outcomes.
Transactional Data: Purchase history, online interactions, and customer service interactions all fall under this category.
Demographic Data: Information like age, gender, occupation, and location.
Third-party Data: External data sources such as market trends, economic indicators, or social media data.
5. Key Elements of Predictive Analytics
Predictive Analytics typically involves five key elements:
Data Collection: Gathering relevant and high-quality data.
Data Analysis: Extracting insights from the collected data.
Statistical Analysis: Using statistical techniques to identify patterns and trends in the data.
Predictive Modeling: Building models that can predict future outcomes.
Deployment and Monitoring: Implementing the models and constantly monitoring and refining them based on new data.
6. Process to Follow for Predictive Analytics
The typical process for predictive analytics involves the following steps:
Problem Definition: Identify the problem or question you want to answer.
Data Collection: Gather the necessary data from various sources.
Data Cleaning & Preprocessing: Prepare the data for analysis by handling missing data, outliers, and irrelevant information.
Exploratory Data Analysis: Understand the structure, trends, and relationships in the data.
Model Building & Validation: Develop predictive models and validate them using techniques like cross-validation.
Deployment: Implement the predictive model into the decision-making process.
Monitoring & Maintenance: Regularly monitor and update the model to ensure its accuracy and relevance.
7. Examples of Predictive Analytics
Netflix’s Recommendation System: Netflix uses predictive analytics to recommend shows and movies to its users. The recommendation engine predicts user preferences based on their viewing history, ratings provided, and behaviors of similar users. This personalized approach has greatly enhanced user engagement and retention for the streaming giant.
Credit Scoring by Financial Institutions: Banks use predictive analytics to determine the creditworthiness of an individual. They analyze a person’s financial history, transaction behavior, and socio-economic indicators to predict the likelihood of future defaults.
Predictive Maintenance in Manufacturing: Companies like GE and Siemens use predictive analytics in their manufacturing units to predict equipment failure. This helps to schedule maintenance activities efficiently, thereby reducing downtime and saving costs.
Fraud Detection in Finance and Insurance: Financial and insurance firms leverage predictive analytics to identify patterns that indicate fraudulent activities. By proactively detecting potential fraud, they can take timely action to prevent substantial losses.
Traffic Management: Predictive analytics has also found use in managing traffic flow in cities. By analyzing historical traffic data and real-time inputs from GPS and IoT devices, cities can forecast congestion points and implement measures to manage traffic effectively.
In conclusion, Predictive Analytics is a powerful tool that allows organizations to be proactive rather than reactive. By using data to anticipate the future, organizations can make more informed decisions, reduce risk, increase operational efficiency, and gain a competitive edge. As more and more data becomes available and machine learning techniques continue to evolve, the potential applications and benefits of predictive analytics are set to grow
Comments